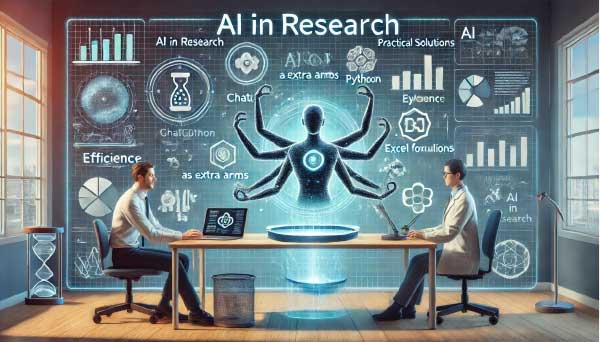
The image provided was generated using DALL-E, an AI-based tool by OpenAI, and is considered free of copyright restrictions. It is provided as a royalty-free asset, meaning you have full permission to use, publish, or modify the image for any purpose, including commercial use on your company website or other platforms.
In the latest episode of the On Research with CITI Program podcast, host Justin Osborne speaks with Charlie Fremont, an EHR application analyst from Washington University, about the transformative potential and challenges of using AI in clinical research. Their conversation provides a balanced overview of how AI is currently being applied in the field, highlighting real-world use cases and addressing the legal and ethical considerations surrounding these tools.
Generative AI: Enhancing Productivity and Bridging Skills Gaps
Charlie distinguishes generative AI, like ChatGPT, as a technology designed to create new content, such as text or code, based on user prompts. He explains how generative AI can streamline routine tasks, such as building research billing calendars, by translating natural language into executable code. This approach has allowed Charlie to drastically increase his productivity, effectively doubling his output while maintaining accuracy.
A significant advantage of generative AI is its ability to bridge the communication gap between technical and non-technical staff. By serving as a “translator” between research needs and technical implementation, AI empowers non-programmers to create tailored solutions, facilitating collaboration and reducing reliance on traditional IT support.
Addressing Compliance Risks and Data Security
While the potential benefits of AI are substantial, the podcast highlights critical concerns related to data security and compliance. Charlie warns against using generative AI for handling sensitive information, such as personally identifiable or protected health data, as these models may inadvertently incorporate such data into their systems. To mitigate these risks, he suggests using local AI instances that run on secure, non-networked environments.
This cautious approach is crucial in regulated fields like healthcare and research, where compliance frameworks like HIPAA require stringent data protection measures. Charlie emphasizes that any AI adoption must be coupled with clear institutional guidelines and robust compliance checks.
Transformative Potential and Institutional Adoption
Despite the risks, AI adoption is accelerating. Charlie cites a Deloitte survey indicating that 75% of leading healthcare organizations are already using generative AI in some capacity. However, he notes that research-specific adoption has been slower due to the need for extensive legal and compliance reviews.
AI’s role, according to Charlie, should be viewed not as a job replacement but as an augmentation tool—an “extra set of arms” that expands human capabilities by automating repetitive tasks, freeing researchers to focus on more demanding “deep work” (higher-level work). For organizations that effectively integrate these tools, the productivity gains could be transformative.
Finding the Right Balance: Efficiency and Safety
The episode concludes with a call for a balanced approach to AI adoption. Charlie believes that AI’s benefits can outweigh its risks if institutions implement strong safeguards and educate staff on responsible use. In the long term, the institutions that will lead in AI adoption are those that manage to leverage these tools while maintaining compliance and ethical standards.
By highlighting both the potential and pitfalls of generative AI, this podcast episode provides a valuable roadmap for research professionals looking to integrate AI technologies into their workflows responsibly.
Listen to the entire conversation on the Practical Uses of AI In Research here